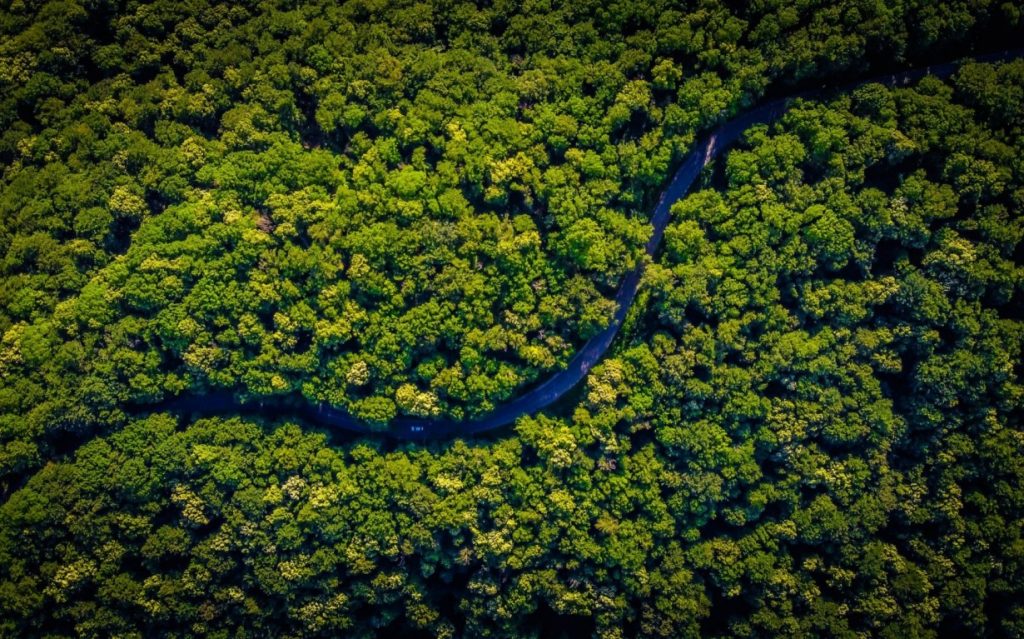
AI And Machine Learning For Sustainability 2.0
AI has the potential to accelerate global efforts to protect the environment and conserve resources by detecting energy emission reductions, CO2 removal, helping develop greener transportation networks, monitoring deforestation, and predicting extreme weather conditions.
Earth5R recently partnered with Eugenie AI, an artificial intelligence software provider based out of India and the US. Shlagha Magon, a team leader at Earth5R, interviewed Dr. Soudip Roy Chowdhury, the Founder and CEO of Eugenie AI and Saurabh Gupta, the founder of Earth5R on the new partnership.
Shlagha: We are really excited about this partnership and we foresee that Eugenie’s technology and the solutions Earth5R co-create together can really amplify the scale and impact of the work that Earth5R does. Today, we’re pleased to be in conversation with Soudip is a PhD-holder in Machine Learning from Trento University in Europe, and headed research at Rakuten and IBM prior to founding Eugenie.
Saurabh Gupta is an environmentalist and social entrepreneur who has also been a project leader at Paris Peace Forum.
My first question is to Soudip.
Soudip, one thing which I personally always found very impressive is your thesis on how the future of industries is going to determine whether or not our world is able to achieve its emissions-reduction targets.
Can you please elaborate your thoughts around this?
Soudip About AI And Machine Learning For Sustainability
Soudip: Hi Shlagha! Thank you for having me here. At Eugenie, we believe that asset reliability and environmental sustainability are two interlinked problems that we can solve using AI. This is because when heavy machinery assets aren’t reliable enough to ensure the desired uptime, backup machinery becomes necessary. This backup adds to overall maintenance costs, carbon footprint and industrial wastage.
Shlagha : That makes sense. Could you tell us a bit about how you got started and what exactly Eugenie does to solve the two interlinked problems you just mentioned…
Soudip: We started Eugenie in 2018 with the vision to make industries more sustainable by using artificial intelligence. What this means is that we use AI-based software that takes real-time data from various sensors connected to heavy machinery and equipment in the oil and gas, metals and mining, smart cities and process manufacturing industries. This software consists of two products – the first is called Ray-Finn and the second is Papillon.
Shlagha: Can you tell us how your products Ray-Finn and Papillon work?
Soudip: Ray-Finn accepts direct machine-level data and checks that the data is as expected to be able to identify any faulty sensors, because if the data is abnormal or inaccurate, then no AI can come up with any useful outputs. Because when it comes to AI, it is Garbage In, Garbage Out.
This verified data is then passed onto our secondary software called Papillon, which analyzes the data using AI models to come up with advance time-bound predictions when the equipment might fail. This means that we can tell operations managers days, weeks and months in advance when their machines may fail, so that they can plan maintenance cycles in an optimized manner.
Shlagha: That’s interesting…so this software presents data to plant operators who can then decide on maintenance prioritization. Is that right?
Soudip: Absolutely! For example, last quarter, we worked with Marc, a plant head who manages a floor of 3,000 interconnected assets.
Due to the nature and scale of his operation, Marc was continuously worried about factors that seemed to be out of his control. These included unscheduled downtimes for seemingly random reasons. Marc was also troubled by rising maintenance costs and emissions. The combined effect of this was that Marc never felt truly in control of his operations.
We went in and used AI to assign risk scores to all the 3000 machines by calculating their failure probabilities while accounting for unique variables such as vibration levels of surrounding machines, floor temperature, etc. By risk-profiling all his assets, we helped Marc to stop worrying about the 99% of equipment that was unlikely to fail before its next maintenance cycle. This empowered him to focus on optimizing maintenance for the 1% of critical equipment that was most at risk.
Shlagha: That’s very impressive. I believe that this type of Artificial Intelligence-based prediction software is also useful for SMART CITY APPLICATIONS. Can you also tell us a bit about how what you’re building is different from any substitute AI or condition-monitoring software in the market?
Soudip: These two Saas products are built on an Explainable AI framework which means that…We track the performance of heavy machinery assets in real time.
This tracking enables us to predict future failures and present our findings in an easy-to-understand manner
When a future failure is deemed probable, we go a level deeper and also figure out the root cause of such a prediction. That way, the human decision-maker who has to take action because of our prediction, has confidence in our system’s recommendation.
But the best part about all this is that we don’t just stop here. We go a step further…And even prescribe next steps with evidence so that the operations team can reduce the likelihood of the failure. This makes Eugenie a trusted ally to plant heads like Marc.
Shlagha: Wow, that’s great. So explainable AI is your key differentiator.
Soudip: Definitely. Eugenie’s Explainable AI includes descriptive and prescriptive insights, generated in a simple and clear language, which can be easily understood by the system users. This helps in establishing the trust of the users and increased awareness.
Most machine learning applications are extremely complex and the results derived from them are something called the “Black box” approach – which means it is impossible to interpret how a particular output is obtained. At times, not even the data scientists and engineers who created the machine learning algorithms can exactly explain how it achieved a specific result.
Thus, turning the voluminous industrial data into actionable information is a humongous task – but Eugenie makes it easy for the industrial companies with Explainable AI. These insights are delivered directly to those who can interpret the information, thereby improving day-to-day decision-making.
Other than explainable AI, our USP is also our ability to achieve rapid forward deployment which enables the Marcs of the world to witness benefits from Eugenie within days, not months or years. Unlike our competitors, you get ROI from Eugenie within the first week of deployment.
Shlagha: You mentioned decision-making. Can you explain the impact of correct and timely decision-making in the process industries?
Soudip: Capturing the operational events with time-series analytics can shed new light on the industrial production processes. Contextual information may reside in various data silos, which can help companies in shifting performance drastically.
Explainable AI enables subject matter experts to contribute to overall organisational performance objectives, such as improved overall equipment effectiveness, reduced wastage, consistent quality, reduction of carbon footprint, and increased operational safety.
Eugenie enables process organisations to gain Operational Excellence and increase overall profitability continuously. We help customers by giving them the answers they need.
Shlagha: That’s interesting. Would you tell us how exactly Eugenie helps companies in achieving operational performance?
Soudip: Eugenie’s plug-and-play solution is easy to use and starts adding value immediately after deployment. It analyses high-volume, time-series industrial data – but it is designed to be used by non-technical operational staff. Captured data through different sources such as SCADA are automatically visualised in the form of user-friendly, graphical representations. Users get detailed root cause recommendations and can monitor performance in real-time to make better faster and better decisions.
Shlagha: Have you faced any challenges in implementing the Explainable AI?
Soudip: The main challenges are Data Silos and the lack of a holistic picture. Many industrial companies install off-the-shelf sensors on their existing machines. Often, they discover that data collected from such sensors is not actually predictive of the failures or performance declines.
In a lot of cases, data-based solutions can identify that there is a problem, but not exactly what the problem is or how to fix it. For truly achieving asset reliability, actionable insights are needed, not just a new set of alarms.
Companies often focus on reducing repair costs or downtime costs. While it is important to provide a short-term business benefit, the biggest opportunities for savings are often less obvious and involves longer-term investment.
The Explainable AI approach can provide many opportunities to improve productivity and profitability. However, Not every such opportunity can be quantifiable at the initial stage.
Shlagha: When it comes to sustainability, what is the level of awareness you have observed in the process industries such as oil and gas?
Soudip: Oil and gas operations are not inherently eco-friendly, however, more and more companies of the sector are investing in research and innovations in various areas such as preventing methane leaks, water recycling, usage of renewable energy, and process efficiency improvements. O&G companies must develop transparent sustainability strategies to transition to a low carbon economy.
Long-term sustainability is not just an option, but an obligatory requirement from investors, customers, and governments. Carbon taxes are a stark reality for many industries, including oil and gas. A safer and greener future is a priority for the most progressive industries and oil and gas are no exception.
Shlagha: Thank you, Soudip for sharing that valuable information with us.Now I would like to ask Saurabh a few questions about how Earth5R is planning to help companies achieve better environmental performance and reduce their overall Carbon footprint using Artificial Intelligence and data technologies.
So Saurabh, since Earth5R is running the River cleaning project in Mumbai could you tell us how AI can be used for a better impact?
Saurabh About AI And Machine Learning For Sustainability
Saurabh: While taking plastic out of the river we also conduct drone flights to capture the data on flow of plastic waste in the river and river catchment area from where waste enters from residential areas to the river.
AI and machine learning can help us analyse this data for better impact by helping us identify different types of waste so prevention strategies can be designed.
Shlagha: That’s interesting, could you tell us how industries could benefit from the same?
Saurabh: Earth5R can help industries like petrochemicals to reduce their oil spills and leakages from pipelines while collaborating with Eugenie using Artificial Intelligence and machine learning which would be beneficial to both the industry as well as the environment to achieve Sustainability. Similarly there are benefits related to reduction of waste, energy and various environmental footprints for manufacturing, utility services, public services and various sectors and it improves their operational efficiency and ROI.
Shlagha: Since you gave an example of the oil pipelines could you tell us if there are ways that the community could benefit from this as well?
Saurabh: Absolutely, cities around the world have been facing many water leakages from the municipality pipelines which is due to wear and tear and improper monitoring of the water pipeline. In India 35 per cent to 40 percent of water is lost, and glbally 33 percent people don’t have access to clean drinking water. Earth5R and Eugenie are working on making use of AI based technology to help reduce water wastage by predicting such situations which can improve the water access globally . Over that, if any tempering of the water pipeline takes place or if there is a high dose of toxin or chemicals in water, that can be detected as well. AI and Machine Learning will play a very vital role in achieving sustainability.
In India 35 per cent to 40 percent of water is lost, and glbally 33 percent people don’t have access to clean drinking water.
Shlagha: That sounds great. Could you give us some more examples where the use of AI can be beneficial when it comes to a different sector, say, mining?
Saurabh: Surely. Let’s say when the equipment/ machine breaks down,-production takes a hit, costs rise and a critical measure of capital efficiency in mining—overall equipment effectiveness (OEE)—goes down. Moreover, requiring unscheduled maintenance is a big task. So by using AI and machine learning, we can predict and prevent the above from happening by monitoring machine health and reliability well in advance. Another example we can take here is the management of inventory and raw materials at factories.
AI automatically orders the correct amount of raw materials to fulfil manufacturing orders and distributors can merge datasets to predict future demand for products. Enabling these organisations to make well-informed business decisions, reduce waste and increase profit.
Shlagha: Since you mentioned factories, is there any way AI I can be used in terms of reduction in energy consumption?
Saurabh: All big factories have backup machines which automatically start when core machines shut down. This consumes a lot of energy which is supplied continuously even when the backup machine is not in use. With AI we can monitor core machines and predict breakdowns in core machines before they take place. As a result backup machines need not be kept on constant supply thus eliminating their need making it cost efficient and saving energy.
Another example can be- power disruption due to unforeseen shutdown at a power-plant – When we use AI and Machine Learning for sustainability to predict these unforeseen shutdowns, the maintenance operations can take place well in hand before the situation arises rather than after.
Shlagha: Wow, sounds like a complete game changer. Could you tell us how AI is transforming waste management and how Earth5R will be making use of the same?
Saurabh: AI and machine learning are constantly improving processes we rely on to collect, transport, sort, and process all types of waste from medical waste to biohazard waste.
Using IoT sensors ( Internet of Things) in “intelligent bins” for monitoring fullness of trash receptacles through the city allows municipalities to optimize waste collection, routes, times and frequencies.
Another thing is smart sorting, while human workers sort between 30-40 recyclables per minute, AI powered machines can handle up to 160. over that machines can work round the clock.
AI machines can also detect items made from different materials as well as nuances between an item of the same material thus making the segregation process much more efficient. Earth5R plans to enable such cutting edge technologies in its projects for transforming the way we approach the management of other types of waste. AI and Machine Learning for sustainability is a new sector and Earth5R being a business leader in Environmental sustainability and Eugenie being a leader in AI and Machine learning space have a lot to contribute towards United Nations Sustainable Development Goals.
Shlagha: Thank you Soudip and Saurabh for this great interview. It’s wonderful to know about this partnership and how it’ll be beneficial to both mankind and nature in terms of sustainability. Looking forward to holding more such interviews on the same!
-Interview conducted by Shlagha Magon